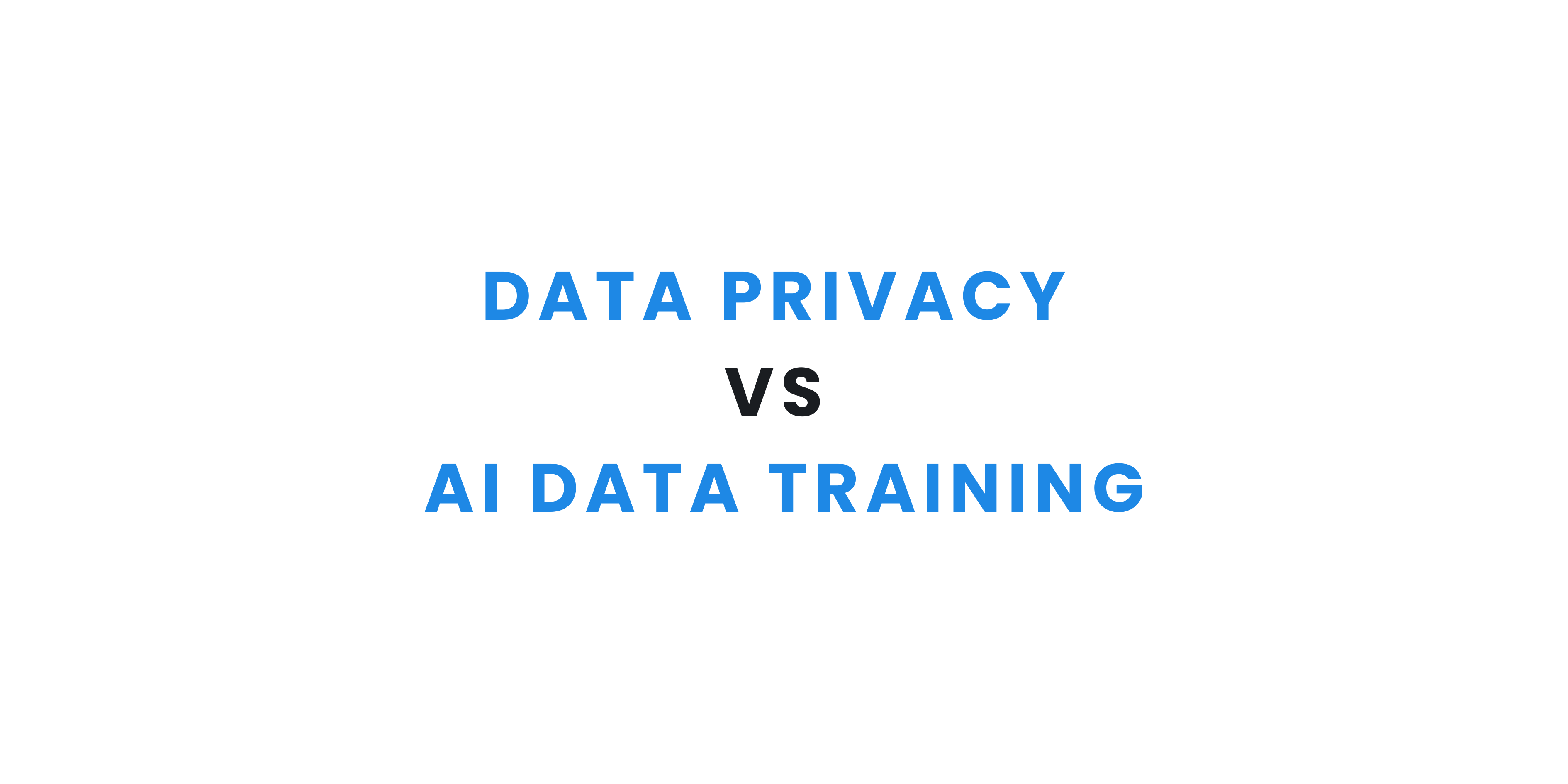
Artificial intelligence (AI) is becoming an integral part of various industries, and more importantly, our daily lives. The importance of data privacy in AI training sets cannot be overlooked. As AI systems rely heavily on vast amounts of data to learn and make decisions, ensuring that this data is handled responsibly and ethically is crucial.
Key Summary
- Data privacy is essential for maintaining individual autonomy and dignity in AI training sets.
- Sensitive personal information in AI training sets must be protected from unauthorised access and misuse.
- Ensuring data privacy builds trust and complies with regulations.
- Challenges include managing large datasets, achieving true anonymisation, and balancing data privacy with AI model fairness.
- Solutions include data minimisation, robust anonymisation, data privacy-preserving algorithms, transparent policies, and federated learning.
The Importance of Data Privacy
Data privacy should be a fundamental human right, essential for maintaining individual autonomy and dignity. When it comes to AI, protecting the privacy of data used in training sets is crucial for several reasons.
Personal Information Protection
AI training sets often contain sensitive personal information. Unauthorised access to or misuse of this data can lead to significant data privacy violations and harm to individuals.
Trust and Transparency
Ensuring data privacy helps build trust between AI developers, users, and the general public. Transparent practices around data usage creates confidence in AI systems and their developers.
Regulatory Compliance
Various laws and regulations, such as the GDPR in Europe and CCPA in California, mandate strict data privacy protections. Compliance with these regulations is not only a legal obligation but also a demonstration of ethical responsibility.
What Are The Challenges?
As artificial intelligence continues to integrate into every aspect of our daily lives, from healthcare and finance to education and entertainment, the volume of data collected and analysed by these systems grows exponentially. The following are some of the key challenges that arise in ensuring data privacy for AI.
Volume and Variety of Data
AI systems require large and diverse datasets to function effectively. Managing data privacy across vast amounts of varied data can be complex and resource-intensive.
Anonymisation Difficulties
Anonymising data to remove personal identifiers is a common practice, but achieving true anonymisation is challenging. Advanced de-anonymisation techniques can sometimes re-identify individuals from anonymised datasets.
Bias and Fairness
Ensuring data privacy while maintaining the quality and fairness of AI models is a delicate balance. Data privacy-preserving techniques must not introduce bias or reduce the effectiveness of the AI system.
The Solutions?
The massive amounts of data required for AI to learn and make predictions pose significant data privacy risks. Therefore, what are the solutions?
Data Minimisation
Collect only the data necessary for the specific AI application. Reducing the amount of personal data collected minimises the risk of privacy breaches.
Anonymisation
Use robust anonymisation and pseudonymisation techniques to protect personal information. Ensure these techniques are regularly updated to counteract advances in re-identification methods.
Data Privacy-Preserving Algorithms
Implement algorithms designed to protect data privacy, such as differential privacy. These algorithms add noise to data in a way that provides statistical guarantees of data privacy without significantly affecting the AI modelโs performance.
Transparent Data Usage Policies
Clearly communicate how data will be used, stored, and protected. Transparency helps build trust and ensures users are aware of their data rights.
User Consent and Control
Obtain explicit consent from individuals before using their data in AI training sets. Provide mechanisms for users to access, modify, or delete their data.
How About Adopting Federated Learning?
Federated learning is an emerging approach that offers a promising solution for data privacy in AI training sets. Instead of sending raw data to a central server, federated learning trains AI models locally on individual devices and only shares model updates. This approach ensures that personal data remains on usersโ devices, significantly enhancing data privacy while still enabling robust AI training.
Conclusion
Data privacy in AI training sets is a critical issue that requires careful consideration and proactive measures. By adopting gold standard practices and leveraging innovative approaches like federated learning, we can ensure that AI systems are developed responsibly and ethically. As we move further into the AI-driven future, prioritising data privacy will be essential for building trustworthy, transparent, and effective AI technologies.