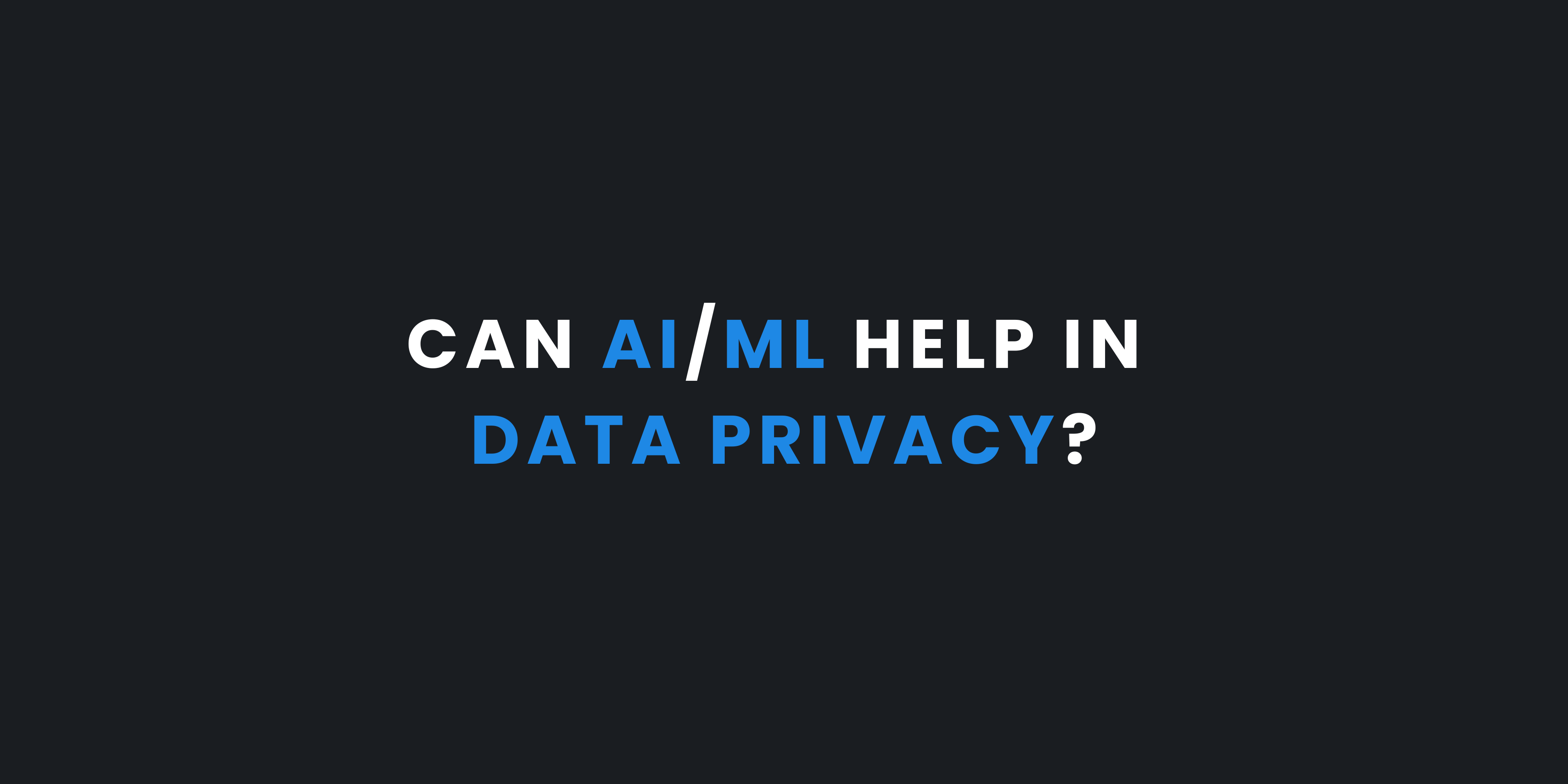
Data is everywhere, and so are the data privacy concerns that come with it. What was once a niche issue is now a major worry for individuals, companies, and governments. Meanwhile, Artificial Intelligence (AI) and Machine Learning (ML) are advancing rapidly, changing industries and our daily lives. So, can AI/ML help with data privacy? Absolutely, though there are some caveats. Let’s explore how these technologies can boost data privacy and what challenges we might face.
Key Summary
- AI and ML can automate anonymisation, detect threats, and facilitate data privacy-preserving data sharing.
- They improve access controls and data minimisation, enhancing data privacy and reducing breach risks.
- Challenges include bias, lack of transparency, data security issues, and regulatory compliance complexity.
- Fair and unbiased AI, explainable models, and secure datasets are crucial for trust in AI-driven data privacy solutions.
- Effective AI/ML for data privacy requires careful implementation and strong ethical considerations.
The Potential
AI and ML have the potential to revolutionise data privacy by providing innovative solutions to protect personal information. These technologies can automate data anonymisation, detect threats in real-time, facilitate data privacy-preserving data sharing, manage access controls, and ensure data minimisation.
Automated Data Anonymisation.
AI and ML can automate the process of data anonymisation, ensuring that personal identifiers are removed from datasets without compromising their utility for analysis. Traditional methods of anonymisation are often labour-intensive and prone to errors. AI-driven algorithms can efficiently detect and mask sensitive information, reducing the risk of re-identification.
Real-Time Threat Detection
Machine learning models can analyse vast amounts of data in real-time to identify unusual patterns or behaviours indicative of a data breach or data privacy violation. By continuously learning from new data, these models can adapt to emerging threats, providing a proactive defence mechanism against cyberattacks.
Data Privacy-Preserving Data Sharing
Federated learning, a newer ML approach, allows models to be trained across multiple decentralised devices (closer to the “edge”) or servers holding local data samples, without exchanging them. This technique ensures that raw data remains on local devices, significantly enhancing privacy while still enabling collaborative learning and analysis.
More Access Controls
AI can help in managing and enforcing access controls by predicting which data should be accessible to which users. Through continuous monitoring and analysis of user behaviour, AI systems can dynamically adjust permissions, ensuring that only authorised personnel have access to sensitive information.
Minimisation of Data
AI can assist in data minimisation by identifying and retaining only the data that is necessary for a specific purpose, thereby reducing the amount of personal information stored. This not only enhances data privacy but also limits the potential impact of data breaches.
The Challanges
While AI and ML offer exciting possibilities for enhancing data privacy, they also come with their own set of challenges and ethical considerations. Issues such as bias in AI models, lack of transparency, data security, and regulatory compliance must be carefully managed to ensure these technologies are used responsibly.
Bias in AI Models
AI and ML models are only as good as the data they are trained on. If the training data contains biases, the models can perpetuate these biases, leading to discriminatory outcomes. Ensuring fair and unbiased AI systems is crucial to prevent worsening of existing data privacy issues.
Transparency
AI decision-making processes can often be opaque or in a “blackbox”, making it difficult to understand how certain conclusions are reached. This lack of transparency can hinder trust and accountability. Developing explainable AI models that provide clear rationales for their decisions is essential for maintaining trust in AI-driven data privacy solutions.
Security
While AI can enhance data privacy, it also requires access to large datasets for training and operation. Ensuring the security of this data is important. Techniques such as differential privacy, which adds noise to data to protect individual identities, can help mitigate this risk, but it is often not enough, and current computing has limitations in enforcing it.
Compliant with Regulations
AI/ML systems must comply with existing data privacy regulations such as GDPR (EU) and CCPA (California). These regulatory pathing can be complex, and ensuring compliance is a continuous challenge.
So, What Lies Ahead?
In conclusion, while AI/ML can significantly enhance data privacy, it is not a silver bullet and an autonomous turret. It requires careful implementation, continuous monitoring, and an unwavering commitment to ethical standards. By exploring how AI/ML can help data privacy, we can better understand the potential and limitations of these technologies and path a future where data privacy is not just an afterthought but a fundamental right.